As of recent, a remarkable barrier has been broken in the world of AI generated artwork: that of the third dimension. Many big players are researching and contributing to this field such as OpenAI, NVIDIA and Google. The models that are currently on the forefront of technical development are already creating promising results but overall seem to still be ever so slightly sub par production levels of quality. However, since AI and ML has been one of the fastest developing technical branches of the recent years, it’s only a matter of time until the models are viable in productions of ever-increasing levels of quality.
Imagine 3D
Luma AI has recently granted limited access to the alpha version of their own text to 3D AI, which both creates 3D meshes as well as textures them.
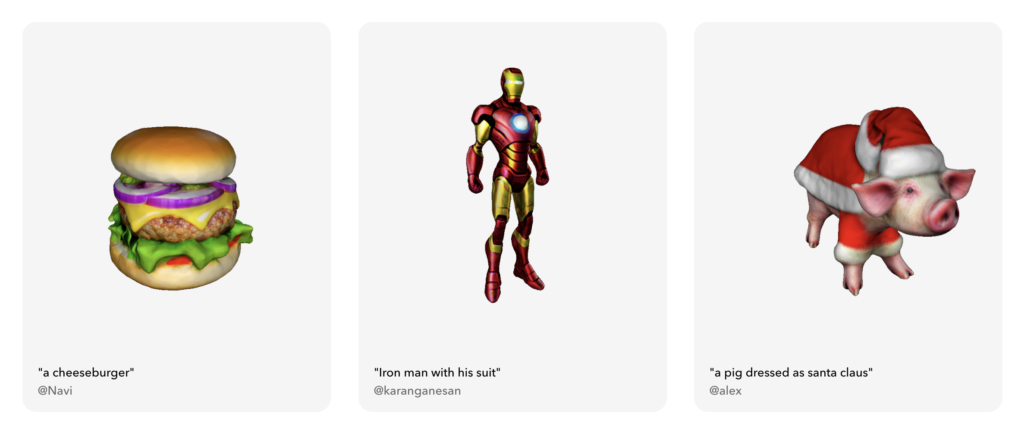
The results look somewhat promising, though upon closer inspection one may notice that the polygon count of the generated models which you can download from their website is quite high while the model itself does not appear to be highly detailed, resembling the results of 3D scans or photogrammetry.
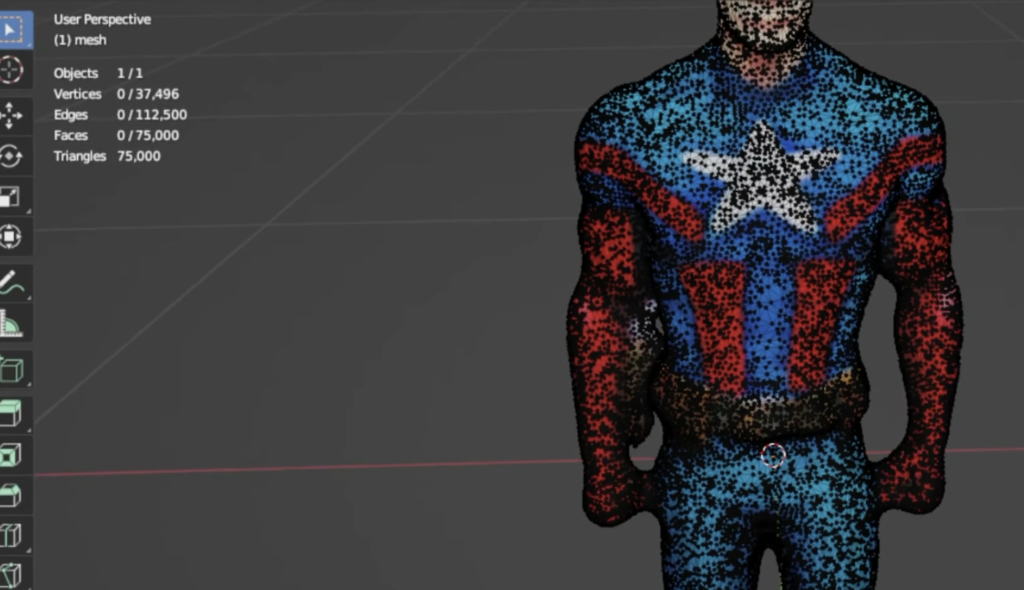
This factor is definitely limiting, however, since photogrammetry and 3D scans have become a common 3D modelling technique in the last few years, there are established ways of cleaning up the results. Bearing in mind that this is the first alpha version of the AI one see great potential in Imagine 3D.
Point-E
As briefly mentioned in my last post, Point-E comes from the same company that created the GPT models as well as the DALL-E versions, OpenAI. Point-E works similarly to DALL-E 2 in that it understands text with the help of GPT-3 and then generates art. The main difference of course being that Point-E works in 3D space. Currently, Point-E creates what you call point clouds rather than meshes, meaning it creates a 3D array of points in space that resemble the text prompt most closely.
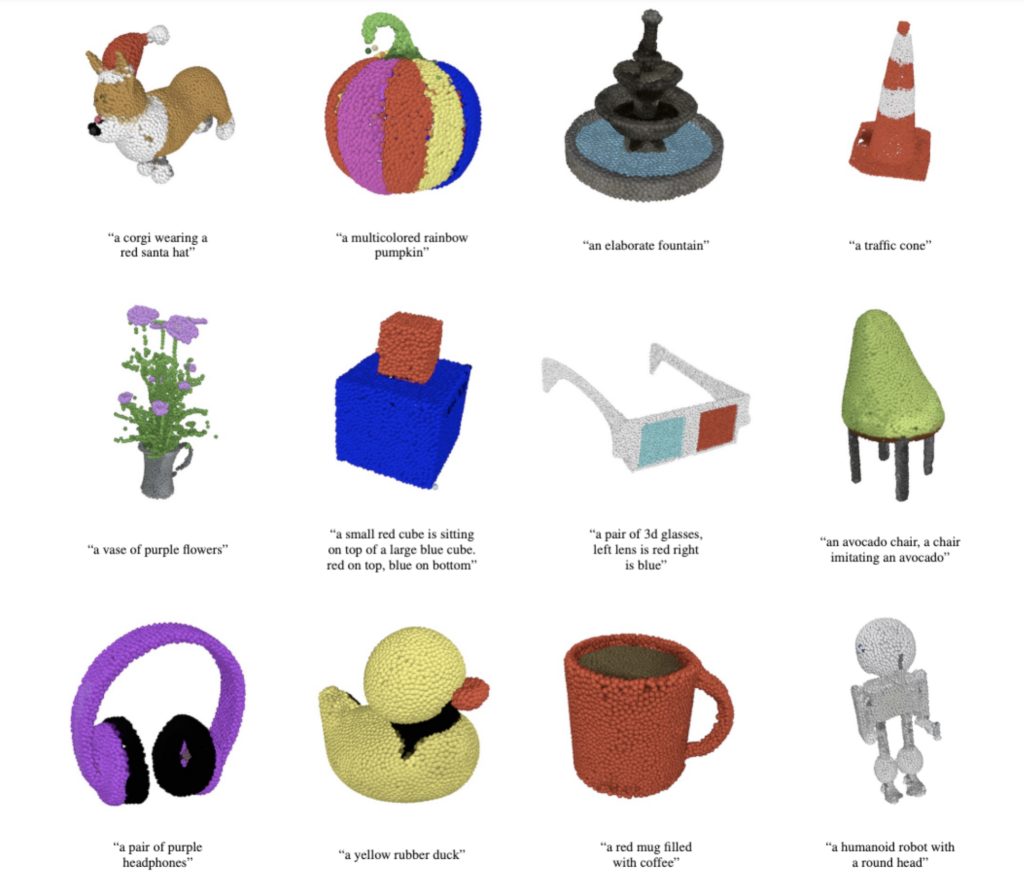
Point-E does not generate textures since point clouds don’t support those, however it assigns a color value to each of those points and OpenAI also offers a point cloud to 3D mesh model that lets users convert their point clouds to meshes.
The left set of images are the colored point clouds with their representative converted 3D mesh counterparts on the right.
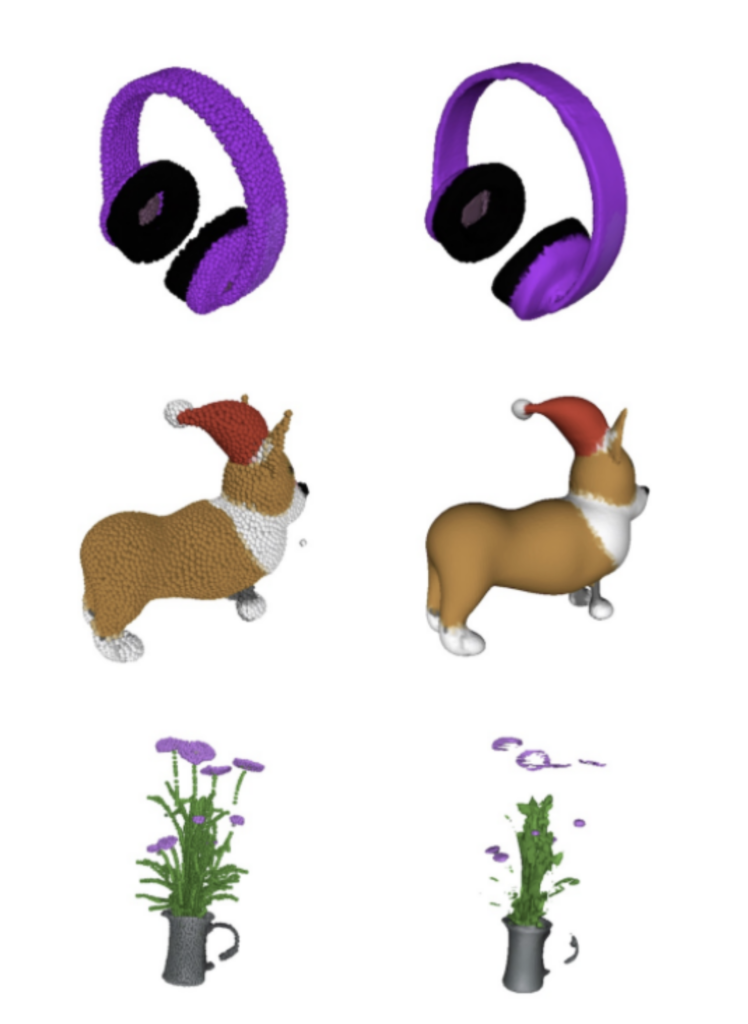
Access to Point-E is limited at the moment, with OpenAI releasing the code on github but not providing users with an interface to use the AI. However, a member of the community has created an environment for users to try out Point-E on huggingface.co.
DreamFusion
Google’s research branch has recently released a paper on their own text to 3D model, DreamFusion. It is based on a diffusion model similar to Stable Diffusion and is capable of generating high-quality 3D meshes that are reportedly optimised enough for production use, but I would need to do more extensive research on that end.
At the moment, DreamFusion seems to be one of the best at understanding qualities and concepts and applying them in 3D space, courtesy of an extensively trained neural network, much like 2D models like DALL-E 2 and Stable Diffusion.
Get3D
Developed by NVIDIA, Get3D represents a major leap forward for text to 3D AI. It too is based on a diffusion model, only that it works in 3D space. It creates high quality meshes as well as textures and offers a set of unique features that, while in early stages of development, show incredible potential of the AI’s use cases and levels of sophistication.
Disentanglement between geometry and texture
As mentioned previously, Get3D generates meshes as well as textures. What makes Get3D unique is its ability to disconnect one from the other. As one can see in the video below, Get3D is able to apply a multitude of textures to the same model and vice versa.
Latent Code Interpolation
One of the most limiting factors and time consuming procedures of 3D asset creating is that of variety. Modelling a single object can be optimised to a certain extent, however, in productions such as video games and films, producing x versions of the same object will, per definition, take x times longer and therefore become more expensive very fast.
Get3D features latent code interpolation, which is a way of describing that the AI understands the generated 3D meshes exceptionally well and is able to smoothly interpolate between multiple versions of the same models. This kind of interpolation is anything but revolutionary, however the implementation of an AI using it to its fullest potential is nothing short of impressive and shows a tremendous level of the AI’s understanding of what it is it is creating.
Text-guided Shape Generation
As text to 3D models are in their infancy at the moment, one of the most challenging tasks is creating stylised results of generic objects, something the 2D text to image models are already excelling at. This means that generating a car is a relatively easy task for an AI, but adding properties such as art styles, looks and qualities gets complex quickly. At the moment, Get3D is one of the most promising models when it comes to this particular challenge, only being outclassed by Google’s DreamFusion, but that is all a matter of personal opinion.