As a big fan of NYU TISCH and their two programs named Interactive Telecommunications Program and Interactive Media Arts, I often search for their teachers GitHub Accounts as many of them provide open access to lecture materials. By doing so they give people who are not studying at this institution the chance of getting in touch with state-of-the-art research for artistic expression.
One of these teachers is Derrick Schultz who not only shares his code and slides but also uploads his entire classes on YouTube. By doing so I was able to follow his Artificial Images class and learn the basics of artificial image creation and state-of-the-art algorithms working under the hood.
Derrick Schultz introduced in his class the following algorithms that are currently used to generate images:
- Style Transfer
- Pix2Pix Model
- Next Frame Prediction (NFP)
- CycleGAN / MUNIT
- StyleGAN
He also gives his opinion on the difficulty of each model and orders them from easiest to hardest.
- Style Transfer
- SinGAN
- NFP
- MUNIT/CycleGAN
- StyleGAN
- Pix2PIX
After comparing the different models, I found two algorithms that could produce the needed video material for my installation.
- Pix2Pix
- StyleGAN
Unfortunately, those are also the ones rated to be the most difficult ones. The difficulty is not only the coding but also data quality and quantity, GPU power and processing time.
In the following section I will analyze the two algorithms and give my opinion whether they could help me generating the visuals for the interactive iceberg texture of my project.
Pix2Pix
As already mentioned, this algorithm can take either image or a video as input and produces according to the training data a fixed output. I could use images of icebergs from NASA or Google Earth as my data set and detect with canny edge algorithm the edges of my images. By doing so, I get from every image in my data set the corresponding edge texture and therefore train the Pix2Pix algorithm to draw iceberg texture by giving edge textures as input.
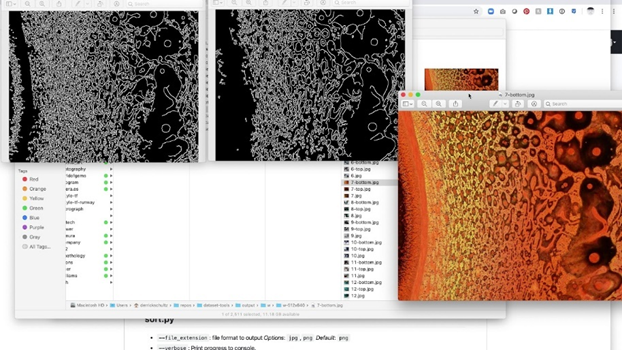
Source: Canny edges for Pix2Pix from the dataset-tools library: https://www.youtube.com/watch?v=1PMRjzd-K8E
Problem:
- The need to develop an algorithm that generates interactive edges.
- Depending on the training set the output can look very different and in the worst case can not be associated with iceberg texture. A lot of training and tweaking results in many iterations of model training.
StyleGAN
In this scenario I could again use iceberg textures from NASA or Google Earth as training data and produce an animation of cracking iceberg texture that shrinks. Since this algorithm produces no fixed output, one can produce endless variations of image material.
A sample animation made by the teacher can be seen here:
Problems:
- Getting in control of the output data of the algorithm is very difficult as it produces random interpolations based on the training data.
- Heavy manipulation of training data might be needed to get the desired outcome. This results in many iterations of model training and therefore a lot of time, computer power, heavy GPU processing and costs.
- Big data set of at least 1000 images recommended.
Link to the Lecture: https://www.youtube.com/@ArtificialImages